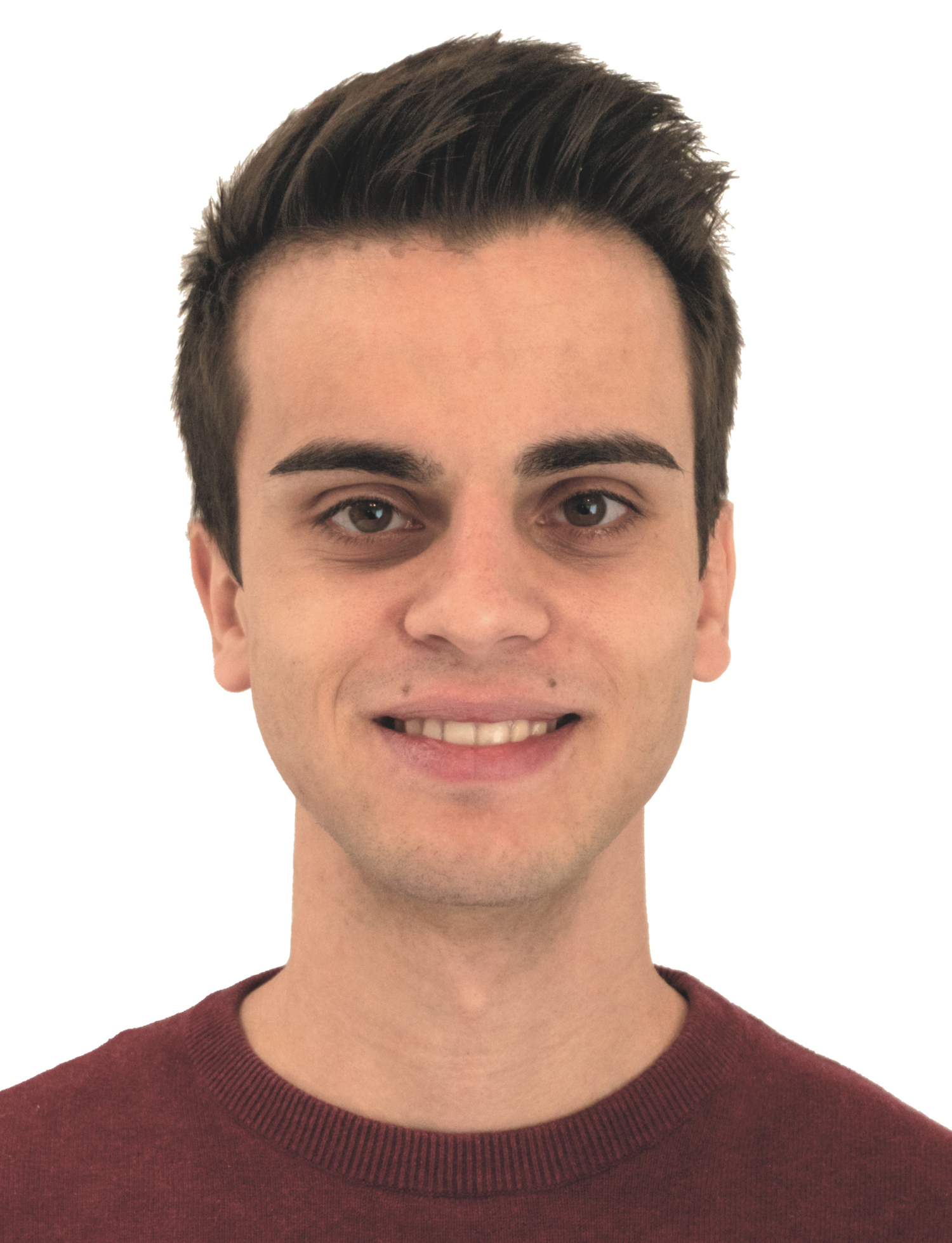
Rafael
Bischof
Personal Website
About me
As a Ph.D. student in the Computational Design Lab at ETH Zurich, my research is focused on utilizing machine learning techniques to tackle engineering challenges by incorporating prior domain knowledge in the form of inductive biases. This results in improved model accuracy and robustness, especially when dealing with limited data in various forms, such as tabular, temporal, image, and graph data. I am particularly interested in physics-informed neural networks, which utilize physical laws and constraints to guide the model’s predictions and enhance performance.
Education
Computational Design Lab - ETHZ
Doctoral Candidate
Eidgenössische technische Hochschule Zürich - ETHZ
M.Sc. in Computer Science with focus on Information Systems
École cole Polytechnique Fédérale de Lausanne ‑ EPFL
B.Sc. in Computer Science
Gymnasium Biel-Seeland
Bilingual Maturität German / French with focus on Physics and Applied Mathematics
Experience
Automation Center - PostFinance
DevOps Engineer, September 2023 – February 2024
Database and application pipeline optimization to enhance efficiency, reliability, and collaboration in software development and database management.
IBM Research
Internship as Machine Learning Engineer, March 2023 – February 2024
Autonomous visual inspection and monitoring of civil infrastructure.
Swiss Data Science Center
Data Scientist, April 2022 – February 2023
Develop and deploy data science and machine learning solutions in collaboration with researchers from various academic disciplines of the ETH Domain.
Research in Orthopedic Computer Science ‑ Balgrist University Hospital
Research Assistant, December 2021 – April 2022
Development of computer‑aided, simulation‑based planning tools as well as the use of Machine Learning and Augmented Reality to support surgeons during the operation, i.e. Development of Unity Apps for Hololens, Reliable & Interpretable ML tools for Health Care.
Institute of Structural Engineering - ETHZ
Research Assistant, August 2021 – December 2021
Study applications of Machine Learning for problems in Civil Engineering, i.e. Physics‑Informed Neural Networks, Generative Adversarial Networks for Beam and Bridge Design, Finite Elements ‑ AI Hybrids for faster inference.
Publications
Multi-objective loss balancing for physics-informed deep learning. arXiv 2021
Strength Lab AI: A Mixture-of-Experts Deep Learning Approach for Limit State Analysis and Design of Monolithic and Laminate Structures made of Glass
The demand for transparent building envelopes, particularly glass facades, is rising in modern architecture. These facades are expected to meet multiple objectives, including aesthetic appeal, durability, quick installation, transparency, and both economic and...
Parametrische Modellierung und generatives tiefes Lernen für den Brückenentwurf
In Anbetracht der erheblichen Umweltauswirkungen des Bauwesens wird die Analyse und v. a. Optimierung der Nachhaltigkeit von Strukturen unter Beibehaltung des etablierten Zuverlässigkeitsniveaus immer wichtiger. Im Hochbausektor existieren erste Werkzeuge zur...